• Goal
- Develop a user-aware, emotion-recognizing model that combines face recognition and representation learning.
- Integrate test-time adaptation for robust performance across various datasets (e.g., Koln, FERV39K, DFEW).
- Optimize the final model for NVIDIA Orin inference, including Docker environment setup, ONNX conversion, quantization, and TensorRT deployment.
- Utilize RADIOv2, a foundation model, to extract robust facial features for downstream tasks.

1.1 Feature Extraction with RADIOv2
- Foundation Model: Leveraged RADIOv2, a pre-trained vision transformer, to extract high-quality facial features: \(F_{\text{RADIOv2}} = f_{\text{RADIOv2}}(I),\) where $I$ represents the input image, and $F_{\text{RADIOv2}}$ is the resulting feature embedding.

1.2 Test-Time Adaptation (Face Recognition)
- Motivation: Achieve stable, adaptive face recognition under domain shifts such as lighting variations, pose changes, and occlusions.
- Approach:
- Researched test-time adaptation methods to fine-tune model parameters during inference: \(W_{\text{new}} = W_{\text{old}} + \eta \cdot \nabla \mathcal{L}_{\text{TTA}},\) where $W_{\text{new}}$ denotes the updated weights, $W_{\text{old}}$ is the original weight, $\eta$ is the adaptation step size, and $\mathcal{L}_{\text{TTA}}$ is the test-time adaptation loss function.

2. Face Recognition + Representation Learning
2.1 Pipeline Design
The pipeline combines RADIOv2, ArcFace, and SimCLR to achieve robust identity verification and emotion recognition.
- Feature Extraction
- Used RADIOv2 to extract foundational facial embeddings:
\(F_{\text{Base}} = f_{\text{RADIOv2}}(I),\) where $I$ is the input image, and $F_{\text{Base}}$ is the extracted feature embedding.
- Used RADIOv2 to extract foundational facial embeddings:
- Representation Learning
- Applied SimCLR for contrastive learning: \(\mathcal{L}_{\text{SimCLR}} = -\log \frac{\exp(\text{sim}(z_i, z_j) / \tau)}{\sum_{k=1}^{N} \exp(\text{sim}(z_i, z_k) / \tau)},\) where $z_i$ and $z_j$ are projections of $F_{\text{Base}}$, $\text{sim}(\cdot)$ represents cosine similarity, $\tau$ is the temperature parameter, and $N$ is the total number of samples.
- Classification (ArcFace)
- Integrated an ArcFace head for face recognition: \(\mathcal{L}_{\text{ArcFace}} = -\log \frac{\exp(s \cdot (\cos(\theta_i + m)))}{\sum_{j=1}^{C} \exp(s \cdot \cos(\theta_j))},\) where $s$ is the scale factor, $\theta_i$ represents the angle between features and weights for class $i$, $m$ is the margin penalty, and $C$ is the number of classes.
- Emotion Recognition Branch
- Added an optional emotion recognition head trained on $F_{\text{Base}}$ for classification tasks: \(\mathcal{L}_{\text{Emotion}} = -\sum_{c=1}^{C} y_c \log \hat{y}_c,\) where $y_c$ is the ground truth for class $c$, and $\hat{y}_c$ is the predicted probability for class $c$.
3. Training & Optimization
3.1 Multi-Teacher Distillation with Loss Formulation
To improve model generalization, the framework utilizes multi-teacher distillation, adapting methods inspired by AM-RADIO:
- Summary Feature Loss
- The student model matches the summary feature vectors of teachers: \(\mathcal{L}_{\text{Summary}}(x) = \sum_{i} \lambda_i \cdot \mathcal{L}_{\text{Cos}}(y_i^{(s)}, z_i^{(t)}),\) where $y_i^{(s)}$ is the student’s summary feature, $z_i^{(t)}$ is the teacher’s summary feature, $\lambda_i$ is the weight for teacher $i$, and $\mathcal{L}_{\text{Cos}}$ is the cosine similarity loss.
- Spatial Feature Loss
- Spatial features of the student are matched to those of the teacher: \(\mathcal{L}_{\text{Spatial}}(x) = \sum_{i} \gamma_i \cdot \big(\alpha \mathcal{L}_{\text{Cos}}(y_i^{(s)}, z_i^{(t)}) + \beta \mathcal{L}_{\text{Smooth-L1}}(y_i^{(s)}, z_i^{(t)}) \big),\) where $\alpha$ and $\beta$ control the weighting of cosine similarity and smooth L1 loss.
- Combined Loss
- The total loss for distillation is: \(\mathcal{L}_{\text{Total}} = \mathcal{L}_{\text{Summary}} + \mathcal{L}_{\text{Spatial}}.\)
3.2 Deployment Optimization
ONNX Conversion
The PyTorch models were converted to ONNX format for hardware-agnostic optimization: \(\text{Model}_{\text{ONNX}} = \text{Export}(\text{Model}_{\text{PyTorch}}),\) where $\text{Model}_{\text{PyTorch}}$ is the original model.
Quantization
Model precision was reduced to INT8 or FP16 to improve latency and reduce memory usage: \(\mathcal{Q}(x) = \frac{\text{round}(x \cdot 2^n)}{2^n},\) where $n$ determines the bit-width of quantization, and $x$ is the original model parameter.
TensorRT Deployment
The TensorRT-optimized model was deployed on NVIDIA Orin, achieving real-time inference with high throughput.
4. NVIDIA Orin Inference & Performance
- Batch Size: 1
- Input Resolution: $224 \times 224$
- Approximate Latency: $15-20$ ms per frame ($\sim 50-65$ FPS).
- Pipeline Integration: Integrated with DeepStream for multi-camera video streaming and real-time analysis.
5. Key Challenges & Solutions
Test-Time Adaptation
- Challenge: Adapting to domain shifts such as lighting changes and occlusions during inference.
- Solution: Implemented test-time loss minimization to dynamically update weights: \(\mathcal{L}_{\text{TTA}} = \| F_{\text{Base}} - F_{\text{Adapted}} \|^2,\) where $F_{\text{Base}}$ represents the original feature embedding, and $F_{\text{Adapted}}$ is the adapted feature embedding.
Representation Learning
- Challenge: Balancing supervised learning (ArcFace) and unsupervised learning (SimCLR).
- Solution: Introduced a weighted multi-task loss to alternate between classification and contrastive learning.
Multi-Teacher Distillation
- Challenge: Combining features from heterogeneous teacher models.
- Solution: Implemented loss balancing with cosine similarity and smooth L1 for effective spatial feature learning: \(\mathcal{L}_{\text{Spatial}} = \alpha \mathcal{L}_{\text{Cos}} + \beta \mathcal{L}_{\text{Smooth-L1}},\) where $\alpha = 0.9$ and $\beta = 0.1$ prioritize cosine similarity.
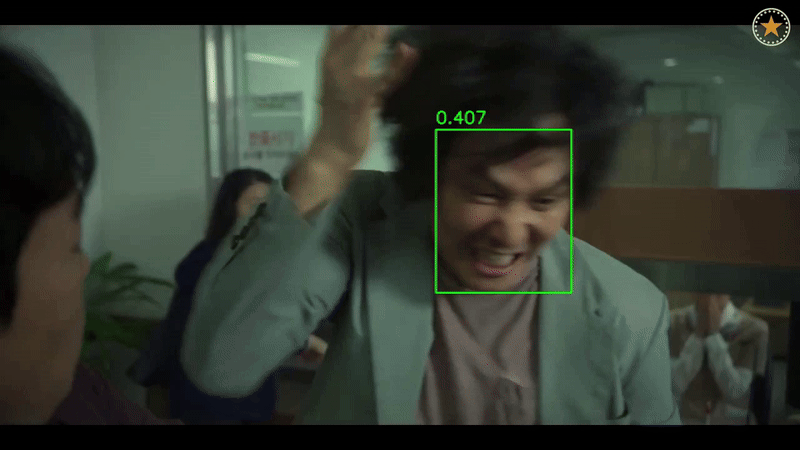
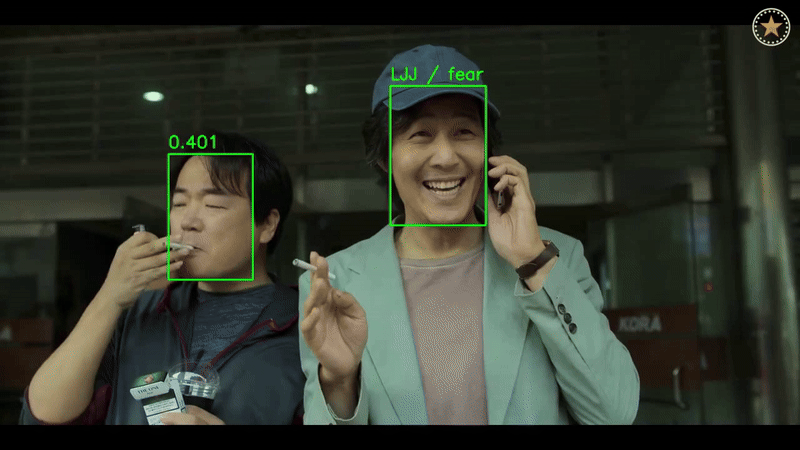
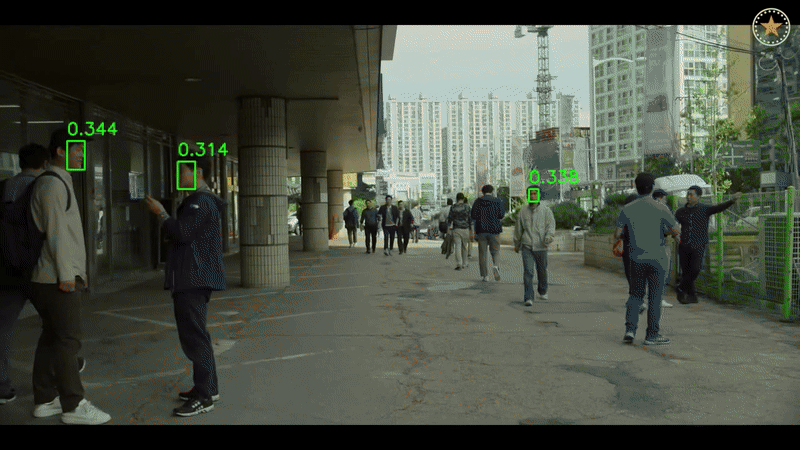
6. Results & Conclusion
Face Recognition
- Achieved state-of-the-art accuracy with ArcFace and RADIOv2 on WebFace and Celeb-1M datasets.
Emotion Recognition
- Demonstrated robust performance on FERV39K and DFEW datasets, showcasing strong generalization across different domains.
Real-Time Feasibility
- Achieved 50-65 FPS inference speed on NVIDIA Orin, enabling real-time emotion detection and face recognition.
Overall
This project highlights the successful integration of RADIOv2, multi-task learning, and hardware optimization. It delivers a robust, real-time solution for face recognition and emotion analysis that performs reliably across diverse conditions and datasets.